Project
Resource T-EXDIZ- Data-supported efficiency increase in the development of textile products through experimentable digital twins using the example of tufting
The development of new tufting products is associated with high material, time and personnel costs. Development times average 6-7 months for known materials and over 12 months for unknown materials.
The aim of this research project is to extend the knowledge gained from the recently completed T-EXDIZ project (IGF 21166N) by parameters for material properties of yarns and carriers. For this purpose, the corresponding material properties (e.g. elongation, carrier type), process parameters (e.g. pile height, needle stroke) and product properties (e.g. basis weight, pile density) will be identified and analyzed for their measurability. These form the basis on which a material data space is subsequently built. This material data space is transformed with expert knowledge into a graphical process model, which represents a quantitative description of all relevant data. With the help of correlation and cluster analysis as well as machine learning methods, correlations and dependencies are determined. The influencing and disturbance variables identified in this process are examined for their data-technical recording (sensor technology, testing, etc.). Subsequently, a categorization takes place according to different criteria (costs, sensor requirements, integration possibilities, etc.), which are then prioritized ("must have", "nice to have", "wish"). The data structured in this way can be included in the data space, which forms the basis for the construction of the digital material twin.
By linking the digital twin of the tufting machine with the digital material twin, the entire tufting process can be virtualized.
In the following step, this AI-supported system will be implemented in an existing business process for technical product development for testing purposes.
The knowledge gained from this test is used on the one hand as a basis for a continuous learning process of the AI and on the other hand evaluated as an assessment basis for the added value through the use of the software.
Finally, a development process of a company is selected and the development effort for a new product is estimated. In the further course, this serves as a reference for the effectiveness of the Experimental Digital Process Twin (EDPZ). For this purpose, the process parameters are transferred to the EDPZ and the development process is carried out with the support of the EDPZ. The data obtained will be examined with regard to expected process reductions and resource savings.
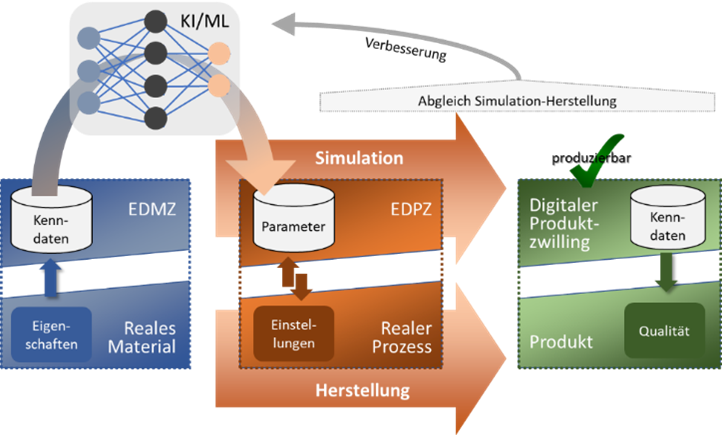
Project partners and/or other research institutes
Forschungseinrichtung 1:
DITF-MR – Zentrum für Management Research der Deutschen Institute für Textil- und Faserforschung Denkendorf
Projektbegleitender Ausschuss:
- Halbmond Teppichwerke Oelsnitz
- Lydall Gerhardi GmbH & Co. KG
- ITA – Institut für Textiltechnik der RWTH Aachen University
- Imbut GmbH
- KARL MAYER Holding GmbH & Co. KG
- Groz-Beckert KG
- HAMMER-IMS nv
- io GmbH
- MMI – Institut für Mensch-Maschine Interaktion der RWTH Aachen
- Vorwerk – Hamelner Teppichwerke GmbH & Co. KG
- Fiberlane GmbH & Co. KG
- Textechno Herbert Stein GmbH & Co. KG
- Freudenberg SE
- OBJECT CARPET GmbH
- codecentric AG
Acknowledgement
The IGF project “Data-supported efficiency increase in the development of textile products through experimentable digital twins using the example of tufting (Resource T-EXDIZ)” 22002 N of the Research Association Forschungskuratorium Textil e.V., Reinhardtstraße 14-16, 10117 Berlin is funded by the Federal Ministry for Economic Affairs and Energy via the AiF within the framework of the program for the promotion of joint industrial research and development (IGF) on the basis of a resolution of the German Bundestag.